Multiplayer AlphaZero – arXiv Vanity
Por um escritor misterioso
Descrição
The AlphaZero algorithm has achieved superhuman performance in two-player, deterministic, zero-sum games where perfect information of the game state is available. This success has been demonstrated in Chess, Shogi, and Go where learning occurs solely through self-play. Many real-world applications (e.g., equity trading) require the consideration of a multiplayer environment. In this work, we suggest novel modifications of the AlphaZero algorithm to support multiplayer environments, and evaluate the approach in two simple 3-player games. Our experiments show that multiplayer AlphaZero learns successfully and consistently outperforms a competing approach: Monte Carlo tree search. These results suggest that our modified AlphaZero can learn effective strategies in multiplayer game scenarios. Our work supports the use of AlphaZero in multiplayer games and suggests future research for more complex environments.

Deep Reinforcement Learning – arXiv Vanity
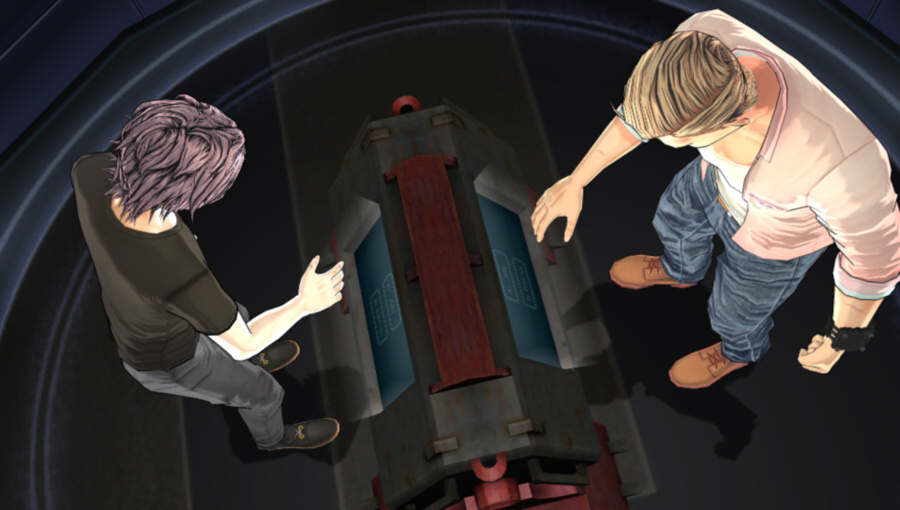
Zero Time Dilemma Part #43 - Ambidex (2 of 2)

Reinforcement Learning Applications – arXiv Vanity

Combining Deep Reinforcement Learning and Search for Imperfect
Overview, Get ready to slay all day in our SlayStation® 2.0 Tabletop and SlayStation Drawers! This bundle features our SlayStation 2.0 tabletop that
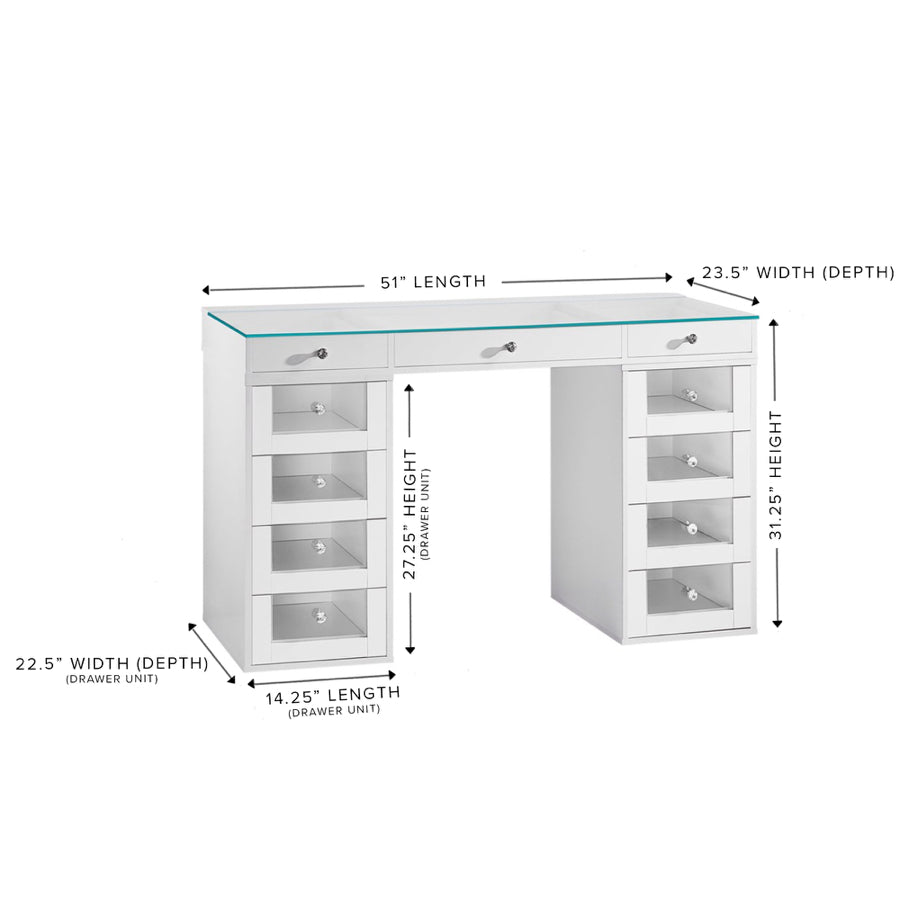
SlayStation 2.0 Tabletop + 4 Drawer Units Bundle

Genius Makers: The Mavericks Who Brought AI to Google, Facebook
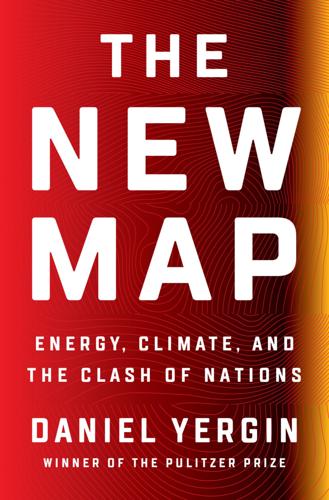
Books: autonomous vehicles
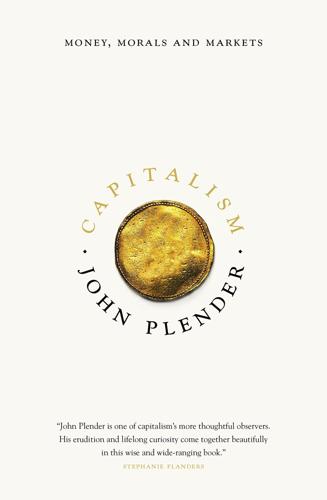
Books: profit motive
Der Hype, die Medien und die Angst
willyb321-stars/README.md at master · jessb321/willyb321-stars

Robots and AI: Our Immortality or Extinction - page 30 - The rest

INTERFACE ZERO 3.0 by David Jarvis/Gun Metal Games — Kickstarter
de
por adulto (o preço varia de acordo com o tamanho do grupo)